Meaningful data is paramount to purpose-driven organizations.
For organizations working toward more equitable and just societies, data is more than numbers and graphs; it’s people, stories and opportunities to change lives and strengthen communities.
In our Data Playbook, Charles and Lynn Schusterman Family Philanthropies lays a framework for how organizations of all kinds can not only capture data more effectively but put it to work underscoring your mission.
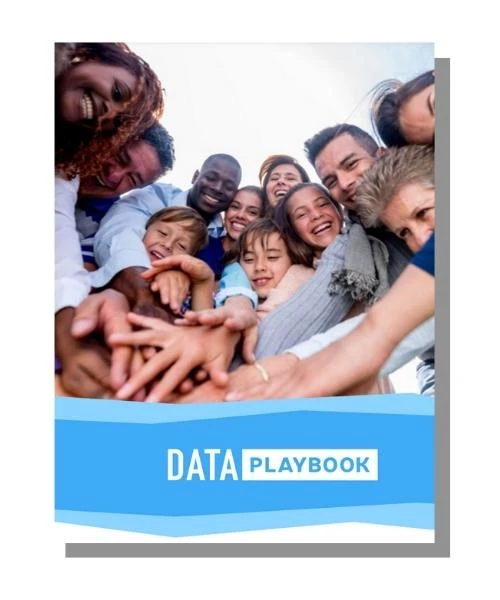
Quote from Rella Kaplowitz
Nonprofit and foundation professionals alike are recognizing data as requisite to becoming stronger stewards of their missions and resources.Playbook Author, Charles and Lynn Schusterman Family Philanthropies
Why It Matters
Surveys found that 94% of nonprofit professionals did not feel that they were using the data at their disposal effectively, and 74% of foundation professionals didn’t feel that evaluations provided any meaningful insights.
We created this interactive resource to help more organizations harness the power of data to make smarter decisions, gain new insights and accelerate progress for the collective good of our communities.
Key Takeaways
This playbook is divided into four core sections:
- What Data to Collect: Why are you collecting and and how does it map to your organization’s strategic goals? It includes tips for defining your key questions, leveraging your strategic plan, refining measurements and setting KPIs.
- How to Collect Data: Get into the nuts and bolts of your data collection strategy, focusing on resourcing, building surveys, setting up interviews and focus groups and obtaining baseline data.
- Making Meaning: After the data collection phase is finished, it’s all about analyzing data. This section provides tips for identifying patterns in data, recognizing trends and setting benchmarks.
- Communicating Results: It’s not just about the results, but how you share them. This section provides helpful insights on making your data tell a story, create impactful visualizations and refine your storytelling over time.
Bonus Content
In the last few pages of the guide, you’ll find links to an incredible array of valuable resources, including articles, books, surveys, focus groups, Excel tutorials, data visualizations and much more.